Authored by BresMed, now part of Lumanity
Therapies designed to target cancers with specific molecular signatures have reshaped the landscape of oncologic drug development.1 As the next generation of tumour-agnostic therapies make their way through clinical trials, we should be asking ourselves what we can do to prepare for the upcoming challenge of reimbursement in this space, and what lessons can be learnt from the experience of existing therapies. Over the coming months, we will provide recommendations from our own experience and a review of the experiences of the tropomyosin receptor kinase (TRK) inhibitors larotrectinib and entrectinib to learn how to overcome the common challenges in this space. Here, in the second of our five-paper series, we focus on the difficulties of estimating the effectiveness of tumour-agnostic treatments in practice.
When considering how effective tumour-agnostic therapies are expected to be in clinical practice, two fundamental issues arise:
- Heterogeneity: will outcomes – e.g. response, progression-free survival (PFS) and overall survival (OS) – differ across patient subgroups (tumour types, or adult versus child)?
- Generalizability: how well do the patients in the trial reflect those expected to be treated in practice?
The two issues are linked. If outcomes are not the same for all types of patients, then the larger the difference between patient characteristics in the trial and in practice, the lower the likelihood that the results of the trial represent those that will be seen in real-world practice. This problem is compounded when, on top of these differences, trial data are immature and there are imperfect surrogacy relationships between measures such as response and survival that must therefore be used to study long-term outcomes.
How can manufacturers tackle such a fundamental challenge when submitting their tumour-agnostic therapies for HTA? Focusing on the recent appraisals of larotrectinib and entrectinib, we look at the roles of heterogeneity and generalizability in recommendation outcomes, the issues that are likely to arise as a result, and the methods and approaches that can be taken to address them. We summarize our recommendations for manufacturers preparing for reimbursement in tumour-agnostic indications at the end of the paper.
Heterogeneity
HTA bodies consistently emphasized heterogeneity when assessing TRK inhibitors
An overview of the appraisals for larotrectinib and entrectinib in tumour-agnostic indications shows that health technology assessment (HTA) bodies consistently emphasized heterogeneity as a barrier to accurately estimating cost-effectiveness. Despite protests from Bayer, the manufacturer of larotrectinib, this view of the underlying data was taken in appraisals of larotrectinib by the Haute Autorité de Santé (HAS), the Gemeinsame Bundesausschuss (G-BA) and the TLV (which also took this view in its appraisal of entrectinib). The uncertainty resulted in conclusions of low clinical benefit for larotrectinib in France and Germany. In Sweden, a perception of a more favourable clinical profile and lower costs in children resulted in a recommendation of larotrectinib for children only and caused concerns in the recent appraisal of entrectinib. In Canada, larotrectinib initially received a negative recommendation, followed by a recommendation with a > 90% price discount at the draft recommendations stage in the recent revised submission.2, 4-8
During the running of the trial, methods can be used to test for heterogeneity of response – either by tumour type or by other relevant characteristics (typically related to the target mutation) – and can inform stopping rules in an adaptive trial design framework. Both frequentist and Bayesian methods are available; these are summarized below.9-11
Frequentist and Bayesian methods
When taking a frequentist approach, either an identical treatment effect is assumed across tumours, or each tumour type is analysed individually. No information is shared between tumour types.
A Bayesian approach, on the other hand, assumes that the estimates of treatment effect of all tumour types can be informed by one another (i.e. exchangeability), but does not assume that treatment effect is identical between them.
Frequentist methods
Easier to identify separate promising baskets
More familiar to reviewers
Less likely to correctly conclude futility or efficacy
The available evidence does not generally support the assumption of homogeneity of drug activity across different tumour types
Bayesian methods
Increases the precision of estimates compared to analysing all baskets separately, whilst reducing the chances of obtaining extreme estimates in baskets with few patients
Recommended in the Experimental Cancer Medicine Centres consensus statement10
Exchangeability assumption aligns with the trial assumption of efficacy related to biomarker rather than tumour site
Only works if borrowing information across baskets is reasonable; an assessment of how reasonable homogeneity of response is should first be conducted
Sensitive to model specification (e.g. priors) when the number of baskets studied is small (< 10)
May unnecessarily increase uncertainty if the response to treatment is homogeneous
Of course, both methods can be used in trial design and analysis. A combination of an initial frequentist assessment followed by a Bayesian approach informed by the result presents a useful strategy to ensure that the ability to share information, which is the major benefit of Bayesian methods, is justified.11 Another benefit of this design is that it allows patient enrolment to be informed by an assessment of the likelihood of homogeneity and by a per-indication assessment of predictive power, based on the observed response and (potentially) the expected response to current standard of care.11, 12 This means enrolment into the trial can be better optimized than it would be if using a more standard enrolment approach.While they are more complex, Bayesian methods are better suited to later HTA because 1) they allow uncertainty to be fully captured in the later economic analysis, and 2) they make best use of all available data to inform estimates.13 Prior beliefs can be informed by earlier-phase trials and/or elicitation of expert input using robust methodologies for capturing quantitative data.
In the latest NICE methods consultation documentation, NICE asks that assumptions about homogeneity, heterogeneity and generalizability of subgroups to clinical practice must be clearly presented, tested and fully explored. It recommends that Bayesian hierarchical models can be used in this context.14
The use of statistical methods to assess heterogeneity of response for the TRK inhibitors demonstrates how the presence of heterogeneity of clinical effectiveness can result in highly variable, uncertain estimates of cost-effectiveness.2, 5, 8, 13, 15
Example of the use of Bayesian Hierarchical Modelling to model the expected distribution of response across tumour types
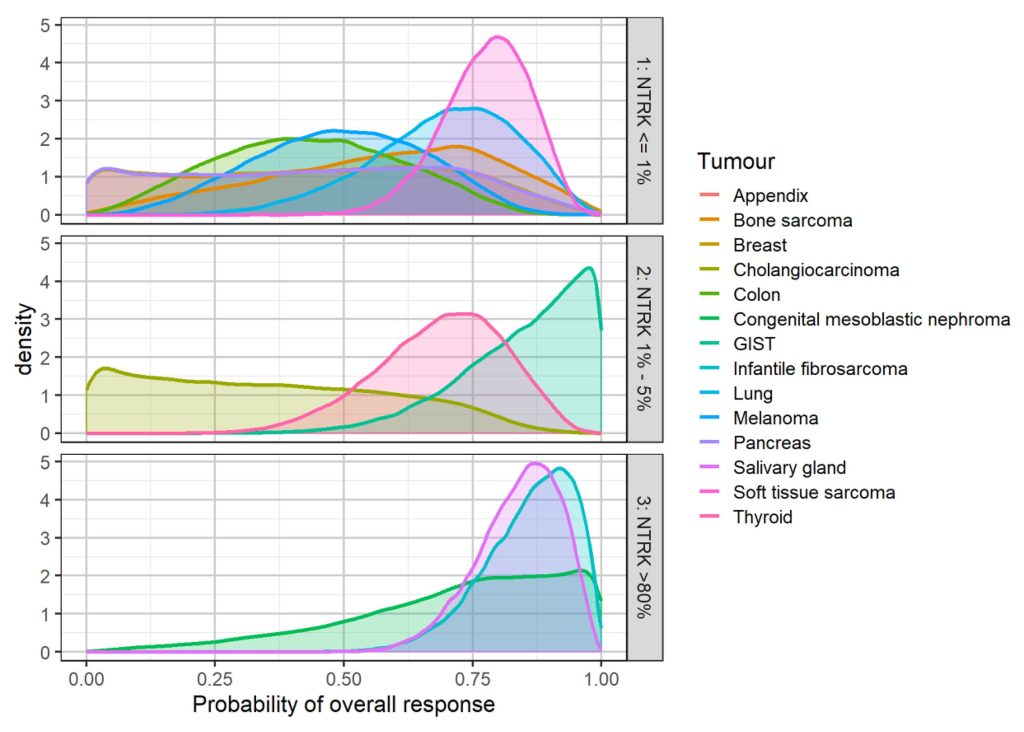
Source: Duarte et al., 2019.16
In theory, Bayesian methods can be applied to both dichotomous outcomes (e.g. response) and time-to-event outcomes (e.g. PFS and OS). However, while the effects of treatment on response can reasonably be assumed to be exchangeable across histologies, it is harder to justify such an assumption on the effects of treatment on survival outcomes. In addition, survival data are often immature at the time of submission13, meaning that outcomes of interest to payers (such as OS) are often predicted based upon surrogate relationships – relationships that are expected to differ by tumour type, and for which little evidence of reliability may exist.17, 18
Generalizability
When appraising tumour-agnostic indications, there may be a number of uncertainties concerning the generalizability of the available evidence, such as9:
- How well do the proportions of tumour types in the trials represent those seen in clinical practice?
- Are there any tumour types that will be encountered in practice that are not represented at all in the trials?
- How well does the therapy’s position in the treatment pathway of the trial reflect the licensed indication and likely practice?
As noted previously, it is extremely unlikely that therapies are equally effective, let alone equally cost-effective, across all tumour types – especially as there are usually different comparators available for each tumour. Given this, where differences between the trial population and the licensed population are considered significant, approaches should be explored that allow for the re-weighting of the trial data so that the data used within the economic analysis better reflect the patients expected to be treated in practice. Such approaches require robust data on the prevalence of biomarkers across different tumour types. As the prevalence of certain biomarkers may vary between different ethnic groups, it could be necessary to collect such data on a per-country basis.
It may be the case – as it is for the TRK inhibitors – that the trial does not collect information on all tumour types within the licensed indication. The impact of this on the generalizability of the trial data will depend both on the size of the missing patient population and on the level of clinical heterogeneity and cost differences across tumour types. Knowing the size of the missing population and using data collection agreements are likely to be the best routes to mitigating this type of uncertainty.
So far, tumour-agnostic licences have only been granted in indications where patients have no other satisfactory treatment options. This is likely to remain the case in the short to medium term. Defining an end-of-line patient population in clinical trials is complicated, however, and trials are therefore likely to have patients enrolled at an earlier stage in their treatment pathway. This is further complicated as the position in the pathway may vary considerably across tumour types according to the availability of alternative treatments. For example, the data used in the TRK inhibitor appraisals were reported from trials of patients at multiple lines of therapy, even in the same tumour type. Line of therapy may be a significant prognostic factor, and failure to adjust for this may substantially affect estimates of effectiveness.
Finally, there is the question of whether limiting these treatments to where there are no satisfactory alternatives is actually the best approach, from a utility-maximizing perspective. If there is variation between tumour types, it seems reasonable to think that earlier positioning of therapies in some tumour types would be a more optimal solution – for example, pembrolizumab’s licence for microsatellite instability-high (MSI-H)/deficient mismatch repair (dMMR) solid tumours includes first-line treatment in metastatic colorectal cancer on the basis of an additional randomized trial. However, this would:
a) require a more robust evidence base than a non-comparative basket trial;
b) potentially undermine the tumour-agnostic approach; and
c) effectively change the mix of eligible patients for later lines to skew it towards those tumour types in which the therapy is less efficacious.
Key points to consider
Avoid assumptions: It is not acceptable to assume homogeneity of response across patients – proving biological rationale for and observation of homogeneity in results is a high bar. It is equally unacceptable to assume that the tumour types observed in trials are generalizable to those seen in clinical practice.
Design trials strategically: The trial design should formally incorporate methods to test for heterogeneity of treatment effect. We recommend Bayesian methods for prediction of treatment effect for final analysis.
Be consistent: Where possible, the statistical methods used for trial analysis and regulatory submission should be propagated through to HTA
Take advice: Seeking joint scientific advice such as EMA-HTA Parallel Consultation is highly recommended for these types of products. Advice should be sought at least 6 months before planned regulatory study initiation19
Plan for uncertainty: Where regulatory strategy is unclear (for example, a focus on promising indications or a full agnostic license), use early economic modelling and landscaping to inform your strategy and plan HTA-focused deliverables to account for the uncertainty
Adapt and adjust: Where trial and practice differ, consider statistical adjustments to present an economic case aligned with practice
Collect data: Data should be collected on current practice for HTA markets in time for submission, such as:
- Prevalence of relevant biomarkers across tumour types
- Prognostic value of the biomarker
- Treatments used and natural history outcomes for patients with the relevant biomarker
- Validity of any surrogates planned to be used in the economic analysis
Use robust expert elicitation: Plan early expert elicitation to inform priors within Bayesian modelling of heterogeneity – robust methods can take considerable time to implement (~9 months)
Propagate uncertainty through your economic model: Uncertainty driven by heterogeneity, generalizability and surrogacy assumptions should be propagated through your economic analysis, just as you would any other source of uncertainty
Be prepared: Additional complexity and analysis may appear during the process, so it pays to be prepared
Publish: You may be able to publish information on current practice and expectations in terms of exchangeability of treatment effect to reference in later HTA submissions
Engage with payers early: Best use of scientific advice mechanisms can help ensure that the right data are collected to supplement clinical trials. An early ‘heads up’ is also extremely important given the volume of work the HTA bodies need to undertake for such indications
Plan data collection after reimbursement: A thorough data collection plan is needed to reduce uncertainties and fill in gaps (such as missing evidence for specific tumour types)
For information on how we can support your next tumour-agnostic therapy, please contact us.
“Consideration of response by tumour location only serves as a distraction and introduces the potential for decision-making to be based on chance findings”
Bayer, NICE appraisal of larotrectinib2
“The high heterogeneity of the study patients and the non-randomized study design complicate the interpretation of important clinical outcome measures … Due to large uncertainties, especially in terms of relative survival, TLV cannot establish a basic scenario regarding cost per quality-adjusted life year (QALY)”
läkemedelsförmånsverket (TLV) appraisal of entrektinib3
“Individual indications have very different cost-effectiveness and budget impact profiles”
Canadian Agency for Drugs and Technologies in Health (CADTH) appraisal of larotrectinib; reappraisal ongoing4
“Response might be different by histology, by NTRK gene fusion or fusion partner, by the presence of co drivers of the disease and by age (for example for children’s indications)”
National Institute for Health and Care Excellence (NICE) appraisals of both larotrectinib and entrectinib2,5
“While the effects of treatment on response can reasonably be assumed to be exchangeable across histologies, it is harder to justify such an assumption on the effects of treatment on survival outcomes.“
“It is extremely unlikely that therapies are equally effective, let alone equally cost-effective, across tumour types“
“So far, tumour-agnostic licences have only been granted in indications where patients have no other satisfactory treatment options“
References
- Offin M, Liu D and Drilon A. Tumor-Agnostic Drug Development. American Society of Clinical Oncology Educational Book. 2018; (38):184-7.
- National Institute for Health and Care Excellence (NICE). [TA630] Larotrectinib for treating NTRK fusion-positive solid tumours. 2020. (Updated: 27 May 2020) Available at: https://www.nice.org.uk/guidance/ta630. Accessed: 31 August 2021.
- Tandvårds- och läkemedelsförmånsverket (TLV). Rozlytrek (entrektinib). 2021. Available at: https://www.tlv.se/download/18.2598f33b179cefa2b4016fc9/
1622807640538/bes210520_rozlytrek_underlag.pdf. Accessed: 31 August 2021. - Canadian Agency for Drugs and Technologies in Health (CADTH). Larotrectinib for Neurotrophic Tyrosine Receptor Kinase (NTRK) Locally Advanced or Metastatic Solid Tumours – Details 2019. (Updated: 10 July 2019) Available at: https://cadth.ca/larotrectinib-neurotrophic-tyrosine-receptor-kinase-ntrk-locally-advanced-or-metastatic-solid. Accessed: 31 August 2021
- National Institute for Health and Care Excellence (NICE). [TA644] Entrectinib for treating NTRK fusion-positive solid tumours. 2020. (Updated: 12 August 2020) Available at: https://www.nice.org.uk/guidance/ta644.
Accessed: 31 August 2021. - Tandvårds- och läkemedelsförmånsverket (TLV). Vitrakvi (larotrektinib) 2020. (Updated: 22 October 2020) Available at: https://www.tlv.se/download/18.7782448f1754f3d6553480b9/
1603884610022/bes201022_beslutsunderlag_vitrakvi.pdf.
Accessed: 31 August 2021. - Canadian Agency for Drugs and Technologies in Health (CADTH). Entrectinib (TBD) for Neurotrophic Tyrosine Receptor Kinase (NTRK) Fusion-Positive Solid Tumours 2020. (Updated: 12 February 2020) Available at: https://www.cadth.ca/entrectinib-tbd-neurotrophic-tyrosine-receptor-kinase-ntrk-fusion-positive-solid-tumours. Accessed: 31 August 2021.
- Canadian Agency for Drugs and Technologies in Health (CADTH). Larotrectinib. 2021. (Updated: 4 May 2021) Available at: https://www.cadth.ca/larotrectinib. Accessed: 31 August 2021.
- Murphy P, Glynn D, Dias S, et al. Modelling approaches for histology-independent cancer drugs to inform NICE appraisals. 2020. (Updated: 28 February 2020) Available at: https://www.nice.org.uk/Media/Default/About/what-we-do/Research-and-development/histology-independent-HTA-report-1.docx. Accessed: 31 August 2021.
- Blagden SP, Billingham L, Brown LC, et al. Effective delivery of Complex Innovative Design (CID) cancer trials-A consensus statement. British journal of cancer. 2020; 122(4):473-82.
- Liu R, Liu Z, Ghadessi M and Vonk R. Increasing the efficiency of oncology basket trials using a Bayesian approach. Contemporary clinical trials. 2017; 63:67-72.
- Ventz S, Barry WT, Parmigiani G and Trippa L. Bayesian response-adaptive designs for basket trials. Biometrics. 2017; 73(3):905-15.
- Murphy P, Claxton L, Hodgson R, et al. Exploring Heterogeneity in Histology-Independent Technologies and the Implications for Cost-Effectiveness. Medical Decision Making. 2021; 41(2):165-78.
- National Institute for Health and Care Excellence (NICE). Review of methods for health technology evaluation programmes: proposals for change. 2021. Available at: https://www.nice.org.uk/about/what-we-do/our-programmes/nice-guidance/chte-methods-and-processes-consultation. Accessed: 31 August 2021.
- Canadian Agency for Drugs and Technologies in Health (CADTH). CADTH Reimbursement Recommendation (Draft): Larotrectinib (Vitrakvi). 2021. (Updated: May 2021) Available at: https://www.cadth.ca/larotrectinib. Accessed: 31 August 2021.
- Duarte A, Corbett M, Grosso A, et al. ERG report: Larotrectinib for treating NTRK fusion-positive advanced solid tumours. 2019. Available at: https://www.nice.org.uk/guidance/ta630/documents/committee-papers. Accessed: 31 August 2021.
- Cooper K, Tappenden P, Cantrell A and Ennis K. A systematic review of meta-analyses assessing the validity of tumour response endpoints as surrogates for progression-free or overall survival in cancer. British journal of cancer. 2020; 123(11):1686-96.
- Chen EY, Haslam A and Prasad V. FDA Acceptance of Surrogate End Points for Cancer Drug Approval: 1992-2019. JAMA Intern Med. 2020; 180(6):912-4.
- Ofori-Asenso R, Hallgreen CE and De Bruin ML. Improving Interactions Between Health Technology Assessment Bodies and Regulatory Agencies: A Systematic Review and Cross-Sectional Survey on Processes, Progress, Outcomes, and Challenges. Frontiers in Medicine. 2020; 7(606).