Authored by BresMed, now part of Lumanity
The market for precision medicine has grown exponentially and continues to grow. Precision medicine considers individual patient characteristics such as their genes, environments and lifestyles to provide tailored treatment options. In this article, we explore the key areas relevant to the economic evaluation of precision medicines and offer practical modelling recommendations based on our experience.
With the growth of precision medicine, examinations of the appropriateness of economic evaluation methods for these innovative therapies are being conducted. From these, a number of key issues when evaluating precision medicines have been identified, as summarized in the figure below.
Key issues that limit the assessment of precision medicine
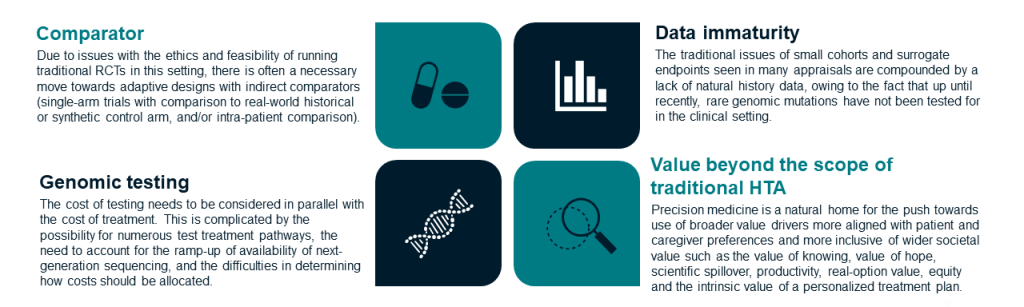
The Health Economics for Personalized Medicine (HEcoPerMed) Consortium has identified challenges associated with modelling health interventions for precision medicine and proposed recommendations on how these challenges should be addressed.1 HEcoPerMed defined precision medicine as ‘a medical model that bases therapeutic choice on the result of gene profiling or aims to correct pathogenic gene mutations’. The study identified eight broad categories of challenges: discounting and perspective, test-treatment pathways, effectiveness data, extrapolating survival, additional elements of value, incorporating compliance, uncertainty analysis and managed entry agreements. While many of the recommendations apply more broadly to health technologies than just to precision medicine, there are some specific considerations that we feel are worth unpacking.
Here, we highlight the recommendations we feel are most relevant regarding differences in testing practices, prognostic markers and additional elements of value, and offer practical modelling considerations.
Capturing the impact of differences in genomic testing practices
Precision medicine requires testing to identify the sub-group of patients for whom a treatment has been designed. Often different tests can be used to identify whether a patient has a certain marker. Also, the settings in which tests are performed can differ, which can affect test performance. Waiting times between taking the test, receiving the result and starting treatment can also vary from setting to setting. Finally, the availability of different tests – and therefore patient access to them – may vary across jurisdictions, along with physician compliance with testing take-up.
If testing in the trial evaluating a new treatment (i.e. the test or tests used, the setting in which tests are performed and waiting times) aligns with testing expected in clinical practice, then budget impact and cost-effectiveness estimates based on models informed using trial data will be reflective of those in clinical practice. If not, adjustments for differences will need to be made.
While adjusting budget impact estimates for differences in test types and performance should be relatively simple, adjusting cost-effectiveness estimates is more complex. One way to quantify the effect of differences in testing practices on cost-effectiveness is to compare the patient and disease characteristics of the trial population with those of the population identified in clinical practice, then combine this information with data on the associations between these characteristics and efficacy, safety and health-related quality of life (HRQL) data from the trial. Ideally, this should be supplemented by targeted literature reviews (TLRs) and expert opinion.
We recommend
- Early TLRs and discussions with experts to determine whether testing practices in countries of interest will align with testing in the trial
- Early TLRs and discussions with experts to determine how differences in testing practices are likely to affect budget impact and cost-effectiveness results
Key HEcoPerMed recommendations: ‘When multiple tests are modelled in sequence, consider the interdependence between test results’ ‘If there is a notable risk of increased morbidity or mortality as a result of waiting periods, incorporate in the model the costs and health outcomes due to the waiting periods’ |
Capturing the impact of prognostic markers
Comparative effectiveness estimates are essential when determining the additional benefit of a precision medicine. When data come from single-arm trials, or when a controlled trial does not include the most relevant comparator, as is often the case, external data sources become necessary. The natural history of the disease and performance of comparator therapies may not be the same in patients with and without the prognostic marker. Given this, there are two potential routes to providing the comparison necessary to demonstrate the value of a new product:
- The preferred option is to collect comparator data in the form of a synthetic or historical control in patients with the relevant biomarker
- If this is not possible due to rarity of the target prognostic marker, determine the impact of the prognostic marker and whether any association with prognosis is independent from other factors (e.g. increased age or reduced performance status); use this to adjust comparator data from a broader population
For the latter strategy to be possible, all important prognostic factors need to be measured in the trial and (summary) data on these prognostic factors should also be available for the broader population.
We recommend using the guidance produced by England’s National Institute for Health and Care Excellence (NICE) Decision Support Unit (DSU) when selecting methods to estimate comparative effectiveness. This guidance provides practical advice on the use of observational data to inform treatment effectiveness, methods for conducting population-adjusted indirect comparisons and flexible methods for survival analyses.2-4 As these guidelines are not updated in real time, and these areas are under constant development, recent literature should always be checked. For instance, after the publication of the NICE DSU Technical Support Document 18, a simulation study resulted in the recommendation that anchored and unanchored comparisons should move away from matching-adjusted indirect comparison and instead use simulated treatment comparisons or multi-level network meta-regression (ML-NMR) where relevant; larger networks should use ML-NMR.5
Randomized controlled trial (RCT) data and real-world data may also need to be combined, which would require a write-up describing the validity of data and bias adjustment. There is not yet any consensus on the methods that should be used for combining RCT and real-world data, though current proposals include hierarchical modelling or design-adjusted analysis. Model-based network meta-analysis should be investigated for cases where networks are not connected due to different treatment dosing or when a different dose will be used in practice than is used in the trial.6 An alternative is to use data from a previous line of treatment as a historical control.7
In addition to considering how the prognostic marker might affect comparator outcomes, attention should be paid to how this may impact other elements of the cost-effectiveness case, such as quality of life and resource use.
We recommend
- Companies should consider the strategy for handling the impact of the prognostic marker and additional data needed in the early design stages of their evidence generation. Otherwise, they may struggle to highlight the value of their treatments to health technology assessment (HTA) bodies
Key HEcoPerMed recommendations: ‘When effectiveness of the comparator for patients with a specific genetic marker is estimated using external data, account for the prognostic value of the genetic marker and differences in its prevalence across the different data sources’ |
Capturing the impact on relatives and value beyond the scope of traditional HTA
Considering the impact on relatives
Because precision medicine often treats diseases that arise from unique genetic mutations, once a patient tests positive for a disease this might result in the testing of family members, adding additional value and costs. From a modelling perspective, the number of relatives tested should be considered in budget impact analyses. This raises the question of whether the costs and effects of testing and treating relatives should also be included in cost-effectiveness analyses. A key consideration here is whether the costs and outcomes for relatives can be expected to be the same as those for the index patient:
- If so, exclusion from cost-effectiveness analysis may be justified, as these would offset one another
- If, however, relatives are expected to be diagnosed and treated earlier in the course of their disease, and outcomes might therefore differ, inclusion should be considered
The number of relatives considered and the period over which to apportion costs is debatable, with no current industry recommendations. Additionally, data to determine the impact of testing relatives on outcomes are likely to be sparse. The number and type of relatives included should be driven by clinical practice; we recommend engaging with clinical experts to understand likely clinical practice and to fill evidence gaps.
Of course, the prevalence of genetic markers will affect both the predicted and actual testing need in a population, and this will need to be considered as it will impact the overall cost of testing. A TLR should be conducted early on to determine whether additional data collection is needed to capture prevalence data – likely to be necessary for new biomarkers and where prevalence varies across geographies.
Inclusion of additional elements of value
HTA bodies aim to ensure value for money. Value is a broad term that has multiple possible definitions, including the most widely used metric: cost per quality-adjusted life year (QALY). Where the QALY is used, extension of life and effects on quality of life are accounted for in value assessments; this is often limited to the patient’s perspective. However, considering additional value elements may be more important for precision medicines to appropriately incentivize innovation and improve allocation of research funding. A recent analysis of submissions to the US Institute for Clinical and Economic Review found that rare diseases were more likely to have broader value elements cited compared with non-rare diseases.8
One key alternative value framework has been proposed. The ‘value flower’ developed by the International Society for Pharmacoeconomics and Outcomes Research (ISPOR) includes further value elements such as scientific spillover, equity, real-option value and value of hope, among others, in addition to the traditional QALY.9, 10 For precision medicines, these are the key additional elements of value that could be considered for inclusion by HTA bodies.
The value of knowing |
Diagnosis of rare diseases can take several years, meaning that patients and their families attach significant value to receiving a diagnosis. Whilst in general the value of knowing increases with the degree of a patient’s risk aversion by reducing uncertainty, because the value of knowing depends on preferences and risk aversion, there is no simple way to value the information provided by a particular test for a population.10 For those wishing to read more, Lakdawalla et al. describe the Generalized Risk-Adjusted QALY (GRA-QALY), which provides a single index accounting for risk aversion in quality of life.11 |
The value of hope |
The value of hope is significant in precision medicine because treatments are often novel and innovative, providing patients with optimism and encouragement. At the same time, treatment efficacy tends to be uncertain, and this uncertainty can have negative consequences such as disappointment or lost hope, which may lead to disutility. These positive and negative effects can be difficult to quantify in a cost-effectiveness analysis. Current literature has focused on the use of discrete choice experiments involving patients.12, 13 However, the value of hope can feasibly be described qualitatively, where appropriate, and included into reimbursement dossier narratives and overall story telling. |
Scientific spillover |
Scientific spillover – whereby new discoveries, such as new biochemical pathways, benefit other areas of science and thus society – is an important phenomenon. However, its value is almost impossible to define, meaning that incorporating it into economic evaluations is infeasible, not least because it is unclear where the value begins and ends, i.e. whether the value is considered indefinitely. |
Real-option value |
Real-option value relates to therapies that extend patients’ lives and give them hope that an alternative and better treatment will be developed. Again, this is difficult to quantify. Methodological challenges include the perspective used in economic evaluations, how to robustly manage forecasting uncertainty, updates required to cost-effectiveness thresholds to incorporate this, and how to avoid double-counting issues.14 |
Disease severity |
Another alternative framework is the one recently proposed by NICE and already used in Norway and the Netherlands, which places greater weight on QALYs gained in more severe conditions via the calculation of the QALY shortfall.15 The recently proposed Generalized Risk-Adjusted Cost Effectiveness (GRACE) approach allows for diminishing returns to health and would have the same end outcome if implemented; thus, optimal cost-effectiveness thresholds should systematically rise for more severe diseases and fall for milder ones.16 |
Equity |
Equity, as highlighted above, is a key area for precision medicine. Not only does it impact diagnosis but also subsequent access to treatments. This may be further negatively compounded by only people of higher socioeconomic status gaining access to certain treatments, thereby exacerbating any pre-existing health inequalities and diminishing the impact of the real-option value and value of hope in these sub-groups. For instance, in the US and other nations where the private healthcare system is dominant, inequity may be significant as richer patients may be willing to pay for genetic testing and personalization, leaving poorer patients behind. Distributional cost-effectiveness analysis methods can be used to include this consideration within economic modelling. |
The value of personalization |
An additional area of value not considered in the value flower but pertinent to precision medicine is the value patients ascribe to personalization in and of itself. This is the value to patients of not being considered ‘just another patient’, which may be more significant in publicly funded healthcare systems. Indeed, patient preferences differ widely, meaning that attaching a value may be difficult and require distributions to be considered – adding further complexities to cost-effectiveness models. However, with the right data and studies determining the level of societal preference, this could be possible to incorporate. |
Overall, while we agree that it is important to adhere to HTA body guidance when submitting reimbursement dossiers, it is also important to ensure that the full potential value of a treatment is described by manufacturers and placed into context. To achieve this for many precision medicines, it will be crucial that alternative value frameworks are considered as part of the submission, even if only in the narrative.
We recommend
- If relatives of index patients become eligible for genetic testing when the index patients test positive for a specific genetic marker, consider whether costs and outcomes are expected to be the same as for the index patient:
- If so, exclusion from cost-effectiveness analysis may be justified as these would offset one another
- If, however, relatives are expected to be diagnosed and treated earlier in the course of their disease, and outcomes might therefore differ, inclusion should be considered
- A TLR should be conducted early on to determine whether additional data collection is needed to capture prevalence data; this is likely to be necessary for new biomarkers and where prevalence varies across geographies
- It is important that manufacturers describe the full potential value of a treatment and place it into context. To achieve this for many precision medicines, it will be crucial to consider alternative value frameworks
- Patient groups should be involved when considering the areas of greatest value to patients, both early in the development process and later when engaging with reimbursement bodies
- Quantitative incorporation of additional value elements may require substantial additional evidence generation; care should be taken to avoid double-counting value elements, to incorporate negative as well as positive aspects, and to account for heterogeneity in preferences between patients and wider society
Key HEcoPerMed recommendations: ‘When multiple tests are modelled in sequence, consider the interdependence ‘If relatives of index patients become eligible for genetic testing when the index patients test positive for a specific genetic marker, include the costs and health outcomes of testing relatives in the economic evaluation of the index patients’ ‘Only include elements of value recommended by national HTA guidelines in the base case. If additional elements of value are included in a sensitivity analysis, ensure possible elements of negative value are equally considered and included for both the intervention and the comparator’ |
References
- Vellekoop H, Huygens S, Versteegh M, et al. Guidance for the Harmonisation and Improvement of Economic Evaluations of Personalised Medicine. Pharmacoeconomics. 2021; 39(7):771-88.
- Phillippo DM, Ades AE, Dias S, et al. NICE DSU Technical Support Document 18: Methods for population-adjusted indirect comparisons in submission to NICE. 2016. Available at: http://nicedsu.org.uk/wp-content/uploads/2018/08/Population-adjustment-TSD-FINAL-ref-rerun.pdf. Accessed: 6 January 2022.
- Faria R, Hernandez Alava M, Manca A and Wailoo AJ. NICE DSU Technical Support Document 17: The use of observational data to inform estimates of treatment effectiveness for technology appraisal: Methods for comparative individual patient data. 2015. Available at: http://nicedsu.org.uk/wp-content/uploads/2016/03/TSD17-DSU-Observational-data-FINAL.pdf. Accessed: 6 January 2022.
- Rutherford M, Lambert P, Sweeting M, et al. NICE DSU Technical Support Document 21: Flexible Methods for Survival Analysis. 2020. Available at: http://nicedsu.org.uk/wp-content/uploads/2020/11/NICE-DSU-Flex-Surv-TSD-21_Final_alt_text.pdf. Accessed: 6 January 2022.
- Phillippo DM, Dias S, Ades AE and Welton NJ. Assessing the performance of population adjustment methods for anchored indirect comparisons: A simulation study. Stat Med. 2020; 39(30):4885-911.
- Pedder H, Dias S, Bennetts M, et al. Joining the Dots: Linking Disconnected Networks of Evidence Using Dose-Response Model-Based Network Meta-Analysis. Med Decis Making. 2021; 41(2):194-208.
- Hatswell AJ and Sullivan WG. Creating historical controls using data from a previous line of treatment – Two non-standard approaches. Stat Methods Med Res. 2020; 29(6):1563-72.
- Shafrin J, Dennen S, Pednekar P, et al. For which diseases do broader value elements matter most? An evaluation across 20 ICER evidence reports. J Manag Care Spec Pharm. 2021; 27(5):650-9.
- Lakdawalla DN, Doshi JA, Garrison LP, et al. Defining Elements of Value in Health Care—A Health Economics Approach: An ISPOR Special Task Force Report [3]. Value Health. 2018; 21(2):131-9.
- Garrison LP, Jr., Zamora B, Li M and Towse A. Augmenting Cost-Effectiveness Analysis for Uncertainty: The Implications for Value Assessment-Rationale and Empirical Support. J Manag Care Spec Pharm. 2020; 26(4):400-6.
- Lakdawalla DN and Phelps CE. Health technology assessment with risk aversion in health. J Health Econ. 2020; 72:102346-.
- Lakdawalla DN, Romley JA, Sanchez Y, et al. How cancer patients value hope and the implications for cost-effectiveness assessments of high-cost cancer therapies. Health Aff (Millwood). 2012; 31(4):676-82.
- Reed SD, Yang JC, Gonzalez JM and Johnson FR. Quantifying Value of Hope. Value Health. 2021; 24(10):1511-9.
- Fornaro G, Federici C, Rognoni C and Ciani O. Broadening the Concept of Value: A Scoping Review on the Option Value of Medical Technologies. Value Health. 2021; 24(7):1045-58.
- National Institute for Health and Care Excellence (NICE). Methods, processes and topic selection for health technology evaluation: proposals for change. 2021. Available at: https://www.nice.org.uk/about/what-we-do/our-programmes/nice-guidance/chte-methods-and-processes-consultation. Accessed: September 2021.
- Lakdawalla DN and Phelps CE. Health Technology Assessment With Diminishing Returns to Health: The Generalized Risk-Adjusted Cost-Effectiveness (GRACE) Approach. Value Health. 2021; 24(2):244-9.